In late November 2019, Amazon announced it was establishing a new unit in its research center in Tübingen, Germany, a university town in central Baden-Württemberg, about 30 kilometers south of Stuttgart. This research team, or what Amazon calls a "Lablet", is dedicated to open research in artificial intelligence (AI), focusing on long-term challenges related to explainability, causality and how AI systems can comprehend their environments. The Lablet is part of the fourth Amazon research and development center in Germany, along with Berlin, Dresden and Aachen.
Yasser Jadidi is Senior Manager of Amazon AWS AI lab there. Previously, he was Director of AI research at the Bosch Center for AI in Renningen, Baden-Württemberg. We reached out to Jadidi to learn more about how the first few months have gone since the research center was first announced, and about the Lablet’s unique approach to fundamental AI research.
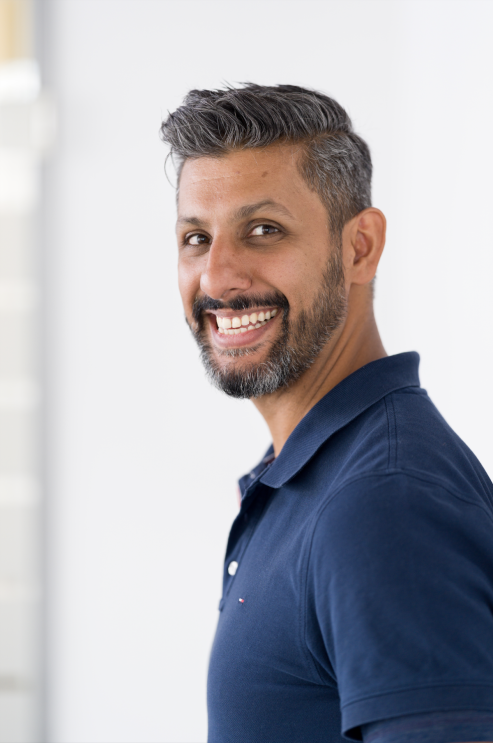
Q. How have the first few months gone since the Lablet was first announced? What’s most surprised you?
A. Actually, it still feels like it is Day One to me, as I am genuinely humbled by both the excellent scientists as well as the competence of candidates that apply for positions here. It is great to build a team rooted in each scientist’s personal curiosity and ambition to solve very hard problems in AI. It’s early days, and I’m only beginning to imagine the scope of ideas that will develop in this setting.
Q. Amazon is known for its applied approach to research. Yet in announcing the Lablet, you indicated the research center would take a more curiosity-driven approach instead of focusing on incrementally improving products and services. Why?
A. We aim at pushing the boundaries of today’s AI capabilities, and at making these capabilities accessible and affordable to society at large. This simultaneously aligns with both our customer orientation and with our responsibility to society.
The access to real-world problems and scalable industrial resources are two essential ingredients we believe are necessary in the field of AI for significant advances. This is exactly the reason for the AWS Lablet; we allow our AI scientists to be exposed to real-world data, domain knowledge and problems, compute power, and the capability to build scalable services that can become accessible to practically everyone in the world.
Q. When announced, you said the Lablet’s research would focus on AI causality and explainability, fairness, privacy, reinforcement learning, and image processing? What do you hope the Lablet can contribute to global research efforts occurring on these topics?
A. First, let me mention that due to the curiosity-driven nature of the research conducted in the Lablet, the actual topics we explore are determined by the scientists themselves.
That said, with the current Lablet setup, I do see a focus around the topics causality, explainability, fairness, and computer vision. Just recently, Thomas Brox, professor of computer vision in Freiburg, joined the Lablet’s list of engaged Scholars, and in mid-April Chris Russell, previously Turing fellow and reader at Surrey in computer vision and machine learning, will join.
Hiring excellent talent to perform self-driven research, exposed to real-world problems and data, and given the right resources, builds a unique setting that can address and solve hard AI problems.
Q. Causality relates to machines understanding the question why. How difficult a research challenge is this? What are the kind of questions you hope to explore related to causal reasoning?
A. Causality is a difficult but very promising AI research field. Causality –not correlation - is the core of human understanding, and both causal discovery, i.e. the extraction of causal patterns from data, as well as inference on determined causal structure, are key to problems related to fairness in AI, explainability of AI-driven systems, or safety of AI-enabled devices.
Our research explores the problems of discovering causal mechanisms from data, of dealing with hidden causes, of disentangling indirect causal influences, and of inferring the rationale behind a system’s behavior from its underlying causal structure. For example, in supply chain logistics, we collect huge amounts of data, but systematic evaluation is difficult due to the high number and variability of parts, demand, suppliers, logistics centers, and observed metrics. The analysis of this data from a causality perspective allows us to understand complex cause-effect relationships, and to find the optimal interventions to avoid delivery delays.
Q. What are the major challenges in building greater trust among the general public in AI systems that are being developed?
A. Building trust for AI systems means establishing familiarity with and predictability of these systems. As AI tools penetrate our daily lives more and more, we need to provide transparency of an AI’s behavior in a way that’s understandable to the general public. Most AI systems today do not focus on explaining their underlying mechanisms, and even if they do, these explanations are for machine learning experts only.
Trust between people is hard to earn and easy to lose. The same is true for AI systems. To build greater trust in AI, the paradigm shift from correlation to causation is key. This is one of the main reasons for our strong focus on causality research.
Q. Your Lablet is collaborating with the Max Planck Institute for Intelligent Systems and the University of Tübingen. Can you explain the model of collaboration you’re developing with those institutions, and how you hope to extend that model to others?
A. We promote open research collaborations with chosen academic partners in our Tübingen Lablet. Our philosophy behind this is to help establish an ecosystem which is mutually beneficial to the academic partners, to Amazon and to society at large. There are many possible aspects to this:
- Research funds such as the Amazon Research Awards, which we provide for instance to the Max Planck Institute for Intelligent Systems, are one element.
- Another element of collaboration are Amazon Scholars, university professors who engage with the Lablet on a part-time basis. This model protects established structures at the university, while providing professors with access to Amazon’s domains and infrastructure.
- Further, Lablet members can collaborate with external academic partners when aiming at shared publications in their field of interest. Moreover, when it comes to publishing research results, we encourage open sourcing related software code to promote transparency and reusability of our results.
- Also, Amazon recently launched its Industrial PhD program in which we enroll outstanding PhD students from collaborating universities, and employ them at the research center in Tübingen. In contrast to classical industry-funded research, PhD students are co-supervised by Amazon employees including our Amazon Scholars and senior research scientists in the Tübingen Lablet. This allows us to reduce additional supervision workload of academic partners. Moreover, we actively encourage university lectures by Amazon researchers to support the education and teaching responsibility of academic partners.